Mastering Data-Driven Product Management: Strategies, Success Stories, and Lessons Learned
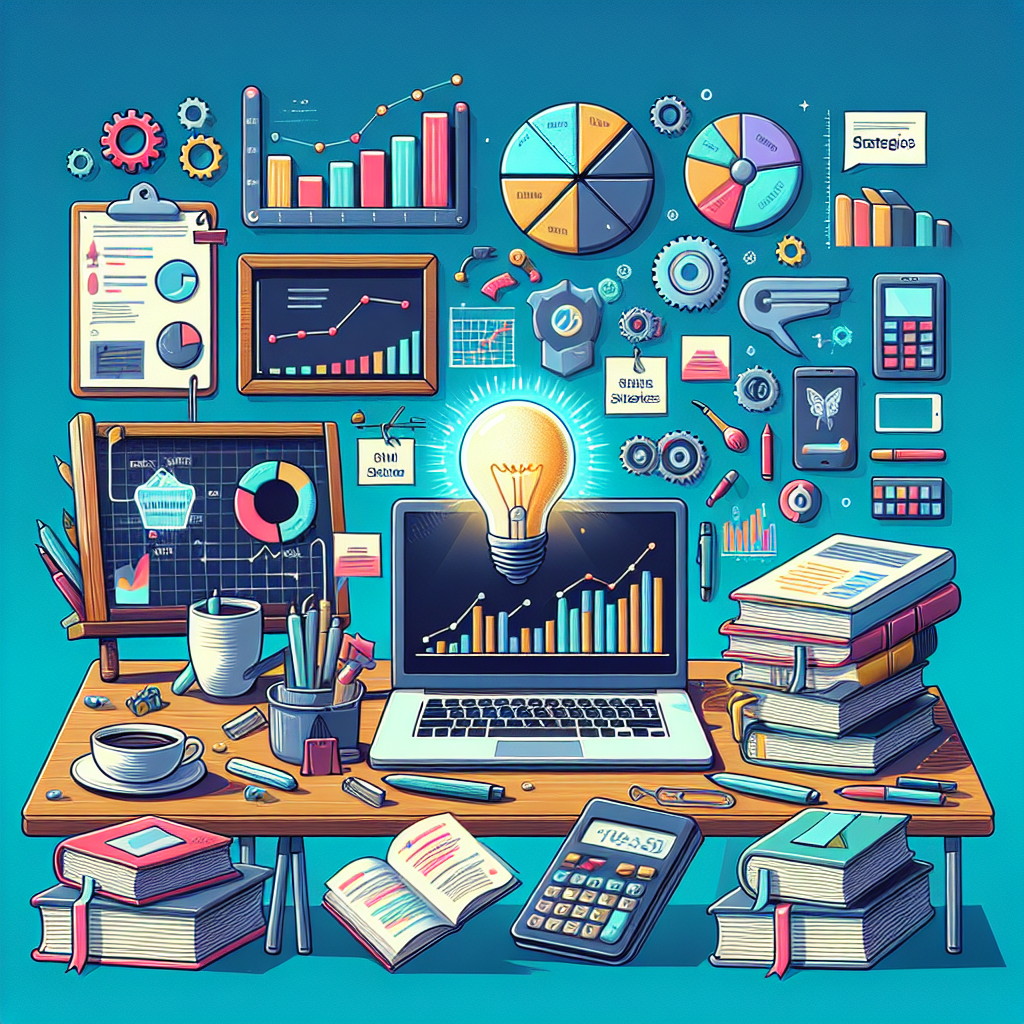
In the complex landscape of modern product management, data-driven decision-making is not just a buzzword—it's a necessity. Product managers must rely on data to validate assumptions, make informed decisions, and optimize their products for success. But how do you navigate the overwhelming volume of data and turn it into actionable insights? In this post, we’ll explore strategies for leveraging data in product management, along with real-world success stories and lessons learned from common pitfalls.
The Importance of Data-Driven Product Management
Data-driven product management involves using quantitative and qualitative data to guide product decisions. The benefits are numerous:
- Optimized User Experience: Understanding user behavior and preferences allows you to create products that meet their needs.
- Informed Decision-Making: Data helps validate or invalidate assumptions, leading to more strategic choices.
- Reduced Risk: By relying on data, you can minimize the risk of product failure and focus on features that deliver value.
- Increased Efficiency: Data enables you to prioritize resources effectively, ensuring that your team works on the most impactful tasks.
Strategies for Leveraging Data
1. Collect the Right Data
Start by identifying the key metrics that align with your product goals, such as user engagement, retention rates, and conversion rates. Use tools like Google Analytics, Mixpanel, or Amplitude to collect and track these metrics.
2. Implement A/B Testing
A/B testing allows you to compare two versions of a feature or design to determine which performs better. Tools like Optimizely and Adobe Target can help you run these tests effectively.
3. Leverage User Feedback
Quantitative data is essential, but qualitative data from user feedback provides context and depth. Use surveys, interviews, and user testing sessions to gather this valuable information.
4. Use Cohort Analysis
Cohort analysis helps you understand user behavior over time. By segmenting users into cohorts based on shared characteristics, you can track how different groups interact with your product and identify patterns.
5. Prioritize Data Visualization
Visualizing data makes it easier to interpret and communicate insights. Use dashboards and visual tools like Tableau and DataStudio to present data in a clear, concise manner.
Success Stories
Case Study: E-commerce Platform Optimization
An e-commerce platform used data-driven strategies to optimize its user experience. By analyzing user behavior, they discovered that a significant drop-off occurred on the checkout page. They implemented A/B testing to identify the best layout and streamlined the checkout process. As a result, their conversion rate increased by 25% within three months.
Case Study: SaaS Product Enhancement
A SaaS company leveraged cohort analysis to improve user retention. They identified that users who completed a specific onboarding tutorial were more likely to remain active. By prioritizing improvements in their onboarding process and encouraging tutorial completion, they boosted their retention rate by 15%.
Lessons Learned from Common Pitfalls
Pitfall: Data Overload
One common challenge is data overload, where teams collect an overwhelming amount of data that becomes difficult to manage and interpret. The lesson here is to focus on key metrics that directly impact your product goals and business objectives.
Pitfall: Ignoring Qualitative Data
Another pitfall is over-reliance on quantitative data while ignoring qualitative insights. Quantitative data tells you what is happening, but qualitative data provides the why. Balancing both types of data gives a more comprehensive view of user behavior and preferences.
Pitfall: Lack of Cross-Functional Collaboration
Effective data-driven strategies require collaboration across teams like marketing, sales, customer support, and development. A siloed approach can lead to fragmented data and missed opportunities. Encourage cross-functional communication and data sharing to ensure holistic insights.
Conclusion
Data-driven product management is key to creating products that resonate with users and achieve business objectives. By collecting the right data, leveraging A/B testing, incorporating user feedback, and using cohort analysis, product managers can make informed decisions that drive success. Learning from common pitfalls further enhances the effectiveness of data-driven strategies. Have you implemented data-driven techniques in your product management process? Share your experiences and insights in the comments below!