AI in Healthcare: Transformative Use Cases, Success Stories, and Challenges
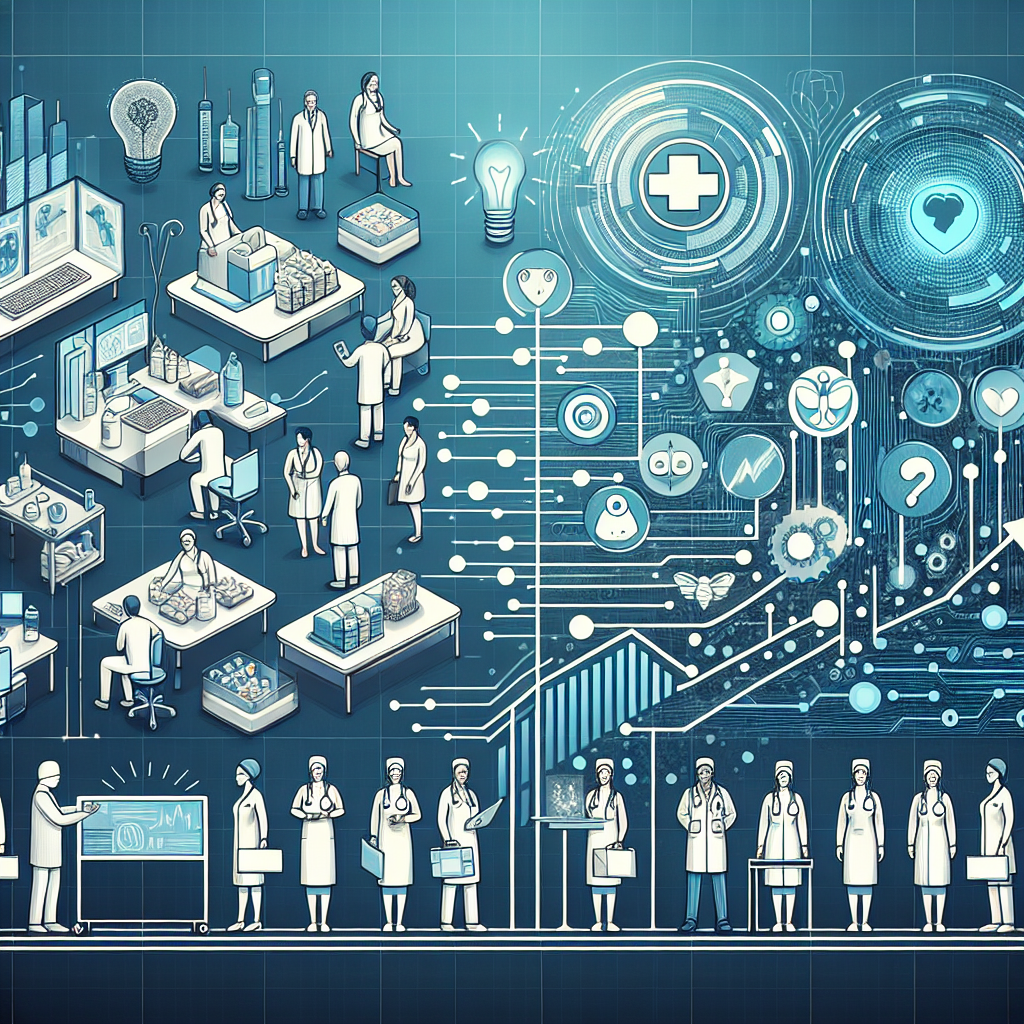
```html
Artificial Intelligence (AI) has radically transformed multiple sectors, and the field of healthcare is witnessing some of the most impactful advancements. From personalized medicine to diagnostics and patient care, AI is revolutionizing how healthcare is delivered. In this post, we explore various AI use cases in healthcare, highlight success stories, and examine lessons from challenges faced during implementation.
AI in Diagnostics and Imaging
AI-powered diagnostic tools are helping healthcare professionals make quicker, more accurate decisions. Machine learning algorithms can analyze medical images to detect abnormalities such as tumors, fractures, and infections. For instance, Google's DeepMind has developed an AI system capable of diagnosing eye diseases by analyzing retinal scans. The system matches or exceeds the performance of human experts.
However, the deployment of AI in diagnostics has its challenges. A significant challenge arose when an AI diagnostic tool showed bias against certain demographic groups, leading to disparities in diagnosis. This emphasized the importance of diverse and representative training data to ensure fair and accurate AI outcomes.
Personalized Medicine
AI is paving the way for personalized medicine by analyzing patient data to tailor treatments to individual needs. IBM's Watson for Oncology, for example, leverages AI to analyze large datasets of medical literature and patient records to recommend personalized treatment plans for cancer patients. This has led to more effective treatment strategies and improved patient outcomes.
Despite its promise, challenges remain. One personalized medicine project faced difficulties when the AI model provided recommendations that conflicted with standard clinical guidelines. This highlighted the need for a collaborative approach where AI serves as a supportive tool for medical professionals, rather than a standalone solution.
AI in Drug Discovery
AI is accelerating the drug discovery process by predicting the efficacy of drug compounds and identifying potential candidates for development. Companies like BenevolentAI use machine learning to parse through scientific literature and biological data, significantly shortening the time required to identify viable drug candidates. This has led to the discovery of new drug compounds that might have otherwise been overlooked.
However, integrating AI into drug discovery is not without its challenges. A pharmaceutical company encountered setbacks when their AI model identified a promising drug candidate that later failed in clinical trials. This illustrated the importance of rigorous validation and the need for AI to complement, not replace, traditional scientific methods.
Patient Monitoring and Care
AI-powered tools are improving patient monitoring and care by analyzing data from wearable devices, electronic health records, and other sources to provide real-time health insights. Hospitals use AI to monitor patient vitals, predict complications, and recommend interventions. For example, the AI system developed by Health at Scale can predict patient deterioration and suggest preventative measures, enhancing patient outcomes.
Despite its benefits, challenges exist in patient monitoring. A health system faced issues when an AI-driven monitoring tool generated numerous false alarms, causing alarm fatigue among healthcare providers. This demonstrated the necessity for AI systems to be finely tuned to balance sensitivity and specificity effectively.
Administrative Efficiency
AI is also enhancing administrative efficiency in healthcare by automating routine tasks such as scheduling, billing, and record-keeping. Natural language processing (NLP) tools are being used to transcribe medical notes, reducing the administrative burden on healthcare providers and allowing more time for patient care. Companies like Nuance provide AI-driven solutions that streamline clinical documentation, leading to increased productivity and reduced burnout among medical staff.
However, implementing AI in administrative tasks can face obstacles. A healthcare network experienced integration issues when their AI scheduling system failed to account for complex variables such as patient preferences and staff availability. This underscored the need for adaptable AI solutions that can handle the nuances of real-world healthcare environments.
The Future of AI in Healthcare
The future of AI in healthcare is bright, with advancements expected in areas such as AI-driven genomics, telemedicine, and mental health support. AI's capabilities in data analysis, predictive modeling, and automation will continue to drive innovation, enhancing the quality of care and patient outcomes.
To fully realize AI's potential in healthcare, it is crucial to address ethical considerations, data privacy, and regulatory compliance. Collaboration between AI developers, healthcare professionals, and policymakers will be key to ensuring the safe, effective, and equitable deployment of AI technologies.
In conclusion, AI is transforming healthcare through applications in diagnostics, personalized medicine, drug discovery, patient monitoring, and administrative efficiency. Success stories from initiatives like Google's DeepMind and IBM's Watson highlight AI's transformative potential, while challenges emphasize the need for continuous refinement and ethical practices. As AI technology evolves, its role in healthcare will expand, offering new opportunities for innovation and efficiency in patient care.
```