AI in Agriculture: Success Stories and Lessons Learned
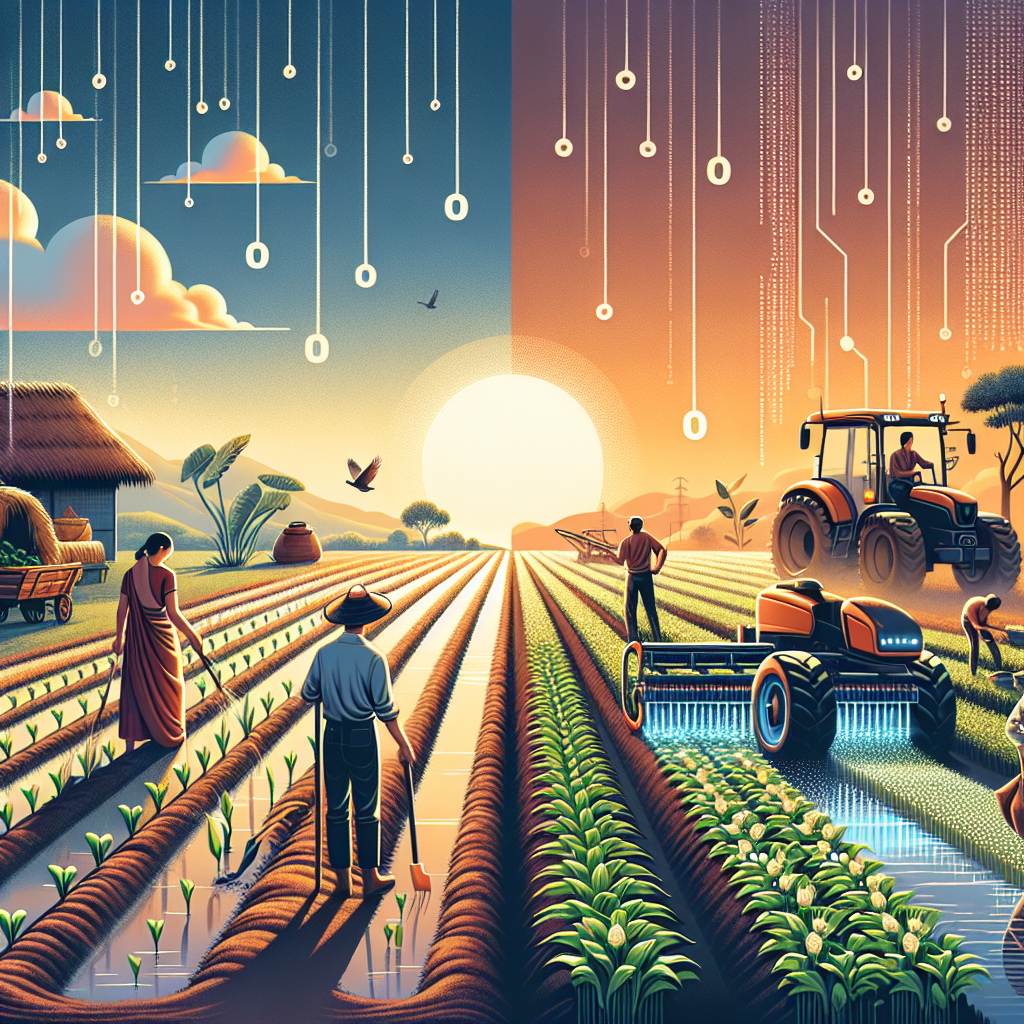
```html
The rapid pace of innovation in Artificial Intelligence (AI) is ushering in a new era for several industries, including agriculture. The global agricultural sector, which faces challenges such as climate change, population growth, and limited natural resources, is increasingly looking to AI for solutions. This post will explore some of the most exciting and impactful use cases for AI in agriculture, sharing success stories and vital lessons learned along the way.
Precision Farming: Optimizing Crop Yields
Precision farming leverages AI to monitor and manage agricultural activities with a level of detail and accuracy that was previously unattainable. Using machine learning algorithms and data from sensors, drones, and satellites, farmers can analyze soil conditions, moisture levels, and crop health. For example, John Deere's AI-driven platform, See & Spray, uses computer vision to identify and treat crops individually, reducing the use of herbicides and optimizing yields.
That said, precision farming is not without its growing pains. There have been instances where AI systems struggled to handle the variability in environmental conditions, leading to suboptimal recommendations. This highlights the importance of continuous data collection and model training to improve accuracy and reliability.
Predictive Analytics: Forecasting Weather and Pests
AI's predictive analytics capabilities are invaluable for anticipating weather conditions, pest invasions, and disease outbreaks. Tools like Climate Corporation's FieldView employ AI to provide farmers with accurate weather forecasts and actionable insights, enabling them to make informed decisions about planting, irrigation, and harvesting.
Despite its potential, predictive analytics must overcome certain challenges. For example, a farming cooperative in Europe faced issues when inaccurate predictions led to overwatering, damaging crops. This incident underscores the need for robust models that can adapt to changing conditions and incorporate diverse data sources for more reliable forecasts.
Automated Harvesting: Increasing Efficiency
Automated harvesting systems equipped with AI technology are transforming the labor-intensive task of crop harvesting. Companies like Abundant Robotics have developed robots that use AI to identify, pick, and sort fruits with precision and speed. These innovations not only reduce labor costs but also minimize crop damage, leading to higher efficiency and productivity.
However, the transition to automated harvesting comes with challenges. Technical malfunctions and the high cost of equipment can be barriers to adoption for smaller farms. For instance, a farm in California faced setbacks when their harvesting robots malfunctioned during peak season, leading to losses. This experience underscores the need for reliable technology and support systems to manage AI equipment effectively.
Supply Chain Optimization: Reducing Waste and Improving Transparency
AI is playing a crucial role in optimizing the agricultural supply chain, reducing waste, and improving transparency from farm to table. Solutions like IBM's Food Trust blockchain leverage AI to trace the journey of produce, ensuring quality and safety. These systems can predict demand and optimize inventory, reducing spoilage and ensuring that fresh products reach consumers.
Nevertheless, integrating AI into the supply chain is a complex task that requires collaboration among various stakeholders. A pilot project in South America faced difficulties when disparate systems and lack of data standardization led to inefficiencies. This example highlights the importance of fostering interoperability and open communication in AI-driven supply chain initiatives.
The Future of AI in Agriculture
The future of AI in agriculture holds immense promise. Innovations in machine learning, robotics, and blockchain technology are poised to drive further advancements in precision farming, predictive analytics, automated harvesting, and supply chain optimization. The potential benefits include increased crop yields, reduced waste, enhanced sustainability, and improved food security.
However, harnessing the full potential of AI in agriculture requires addressing several key challenges. Investing in reliable infrastructure, ensuring data accuracy, and fostering collaboration among stakeholders are essential for successful implementation. Additionally, ethical considerations, such as the impact on employment and data privacy, must be thoughtfully addressed.
In conclusion, AI is revolutionizing agriculture, offering solutions to some of the industry's most pressing challenges. Success stories from companies like John Deere, Climate Corporation, and Abundant Robotics showcase AI's transformative potential. However, lessons learned from challenges and setbacks provide valuable insights for future implementations. As AI continues to evolve, its applications in agriculture will expand, paving the way for a more efficient, sustainable, and resilient global food system.
```